Table of Contents
1. Introduction
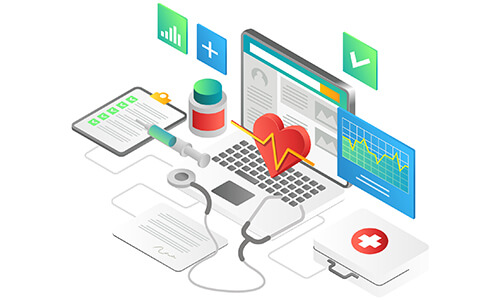
All commercial and non-profit organizations generate business data because of day-to-day operations. Analyzing this data reveals many things about an organization and its operations, even things that are not immediately clear to the outside observer. The bigger the organization, the greater the amount of data generated. The same applies to healthcare.
Healthcare as an industry generates enormous amounts of data regarding insurance, reimbursements, patients’ health, population health, medical billing, chronic and infectious diseases, and so forth. Assessing all this information about day-to-day operations and thousands of patients requires a dedicated technological solution. As the name indicates, healthcare analytics solutions empower the medical industry to harness valuable insights from the data and use them to improve the quality of care.
In addition to clinical care itself, analytics in healthcare also sheds light on the patterns in reimbursements, profitability, care continuum, patient outcomes, the prevalence of diseases, and much more. Knowledge of these helps doctors and administrators make informed decisions about various aspects of healthcare management, which in turn benefits all the stakeholders.
This is why almost every industry has invested more and more resources into implementing data analytics solutions. Whether tourism, aviation, software, real estate, telecommunications, or even hospitality, data has emerged as potent fuel for growth.
2. Types of Data Analytics
In simple words, data analytics, as the name indicates, is the assessment of the data to derive useful conclusions and identify patterns. In healthcare, this involves combing through patients’ medical records, hospitals’ billing procedures, usage of insurance plans, and so forth. But there is more than one way of analyzing data, and in this section, we will explore the different types of data analytics.
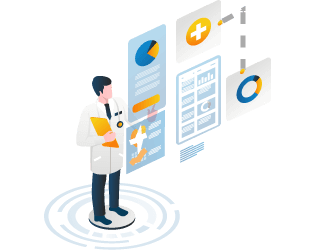
Data analytics has been used by almost every industry, and there have been sustained efforts to develop ever more effective means of analysis. The technology and innovation of analytics aside, when it comes to the actual means of analysis, there are certain types of going about the data. Each of those types yields a separate kind of result, and they are all useful in different ways.
The following are the types of analytics used in healthcare –
1. Descriptive Analytics
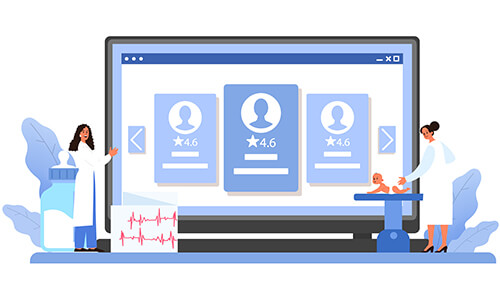
This is perhaps the most rudimentary form of analyzing data, and it talks more about events that have already happened in the past and derives useful patterns from those events. These patterns, in turn, help healthcare providers, administrators, and even government policymakers gain important insight into healthcare. It can be considered the first step towards transforming data into a format that is usable by people and quantifying past events that are on record.
For example, the descriptive analysis highlights things like how many patients were admitted with gunshot wounds in the last year, the number of babies born within a certain space of time, how many patients over a certain age were diagnosed with a certain disease, the number of patients showing symptoms of a disease, and much more. In other words, the descriptive analysis describes what happened.
These patterns not only help doctors manage the care process but also enable policymakers to identify patterns in diseases and frame regulations accordingly.
2. Diagnostic Analysis
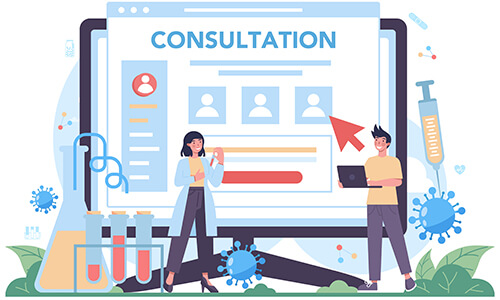
In medical terms, diagnosis means identifying the nature of a problem by investigating the symptoms. While descriptive analysis tells us what happened, diagnostic analysis tells us why. This assessment data is great for classification and finding correlations among statistical information.
For example – if it is revealed that people over a certain age are more prone to diabetes through descriptive analysis, the diagnostic analysis may shed some light on the why of things.
This required a deeper level of data analysis than just mere pattern finding. In the above example, to know why people over a certain age might be more vulnerable to diabetes, the diagnostic analysis might further analyze their habits, lifestyle, diet, and other information to find certain correlations that might point towards the real reason.
3. Predictive Analysis
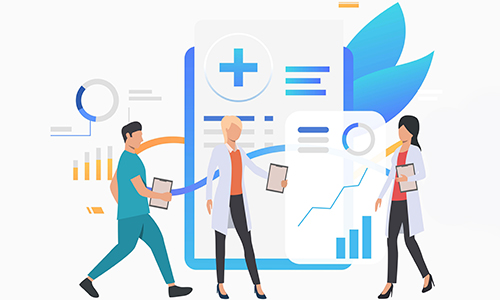
As the name suggests, predictive analysis tries to predict what will happen based on historical and current data. It can be called less of a prediction and more of a deduction of future events based on what has already transpired and what is happening. Many experts think that this would be one of healthcare analytics’ biggest accomplishments and would transform healthcare as a whole.
Numerous investors have been pouring money into promising companies with hopes of developing the best commercial predictive healthcare analytics services solution. Moreover, researchers have also stated that predictive healthcare analytics could help reduce chronic diseases in the United States. Data about a person’s lifestyle, diet, and ancestry, coupled with basic health information and medical history, could help identify if he or she is at higher risk of certain diseases. This would empower doctors to take a proactive approach during the early stages rather than a reactive approach when the disease is full-blown.
4. Prescriptive Analytics
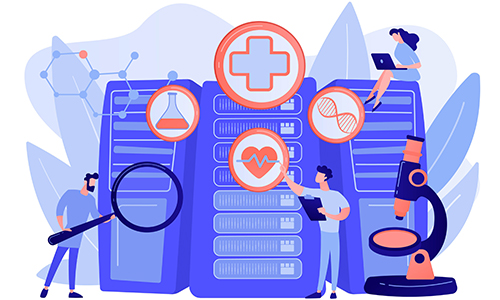
Prescriptive analytics for healthcare acts upon the predictions derived from predictive analysis. Technologists agree that this represents the final stage of analytics. A sick patient is “prescribed” a treatment to improve his or her health. Similarly, when there is a prediction about something that is about to happen, prescriptive analysis helps users to know how to correct the course of events to prevent the outcomes that were predicted or mitigate the ill-effects of the same. In other words, it “prescribes” the necessary action or intervention for managing the risks.
For healthcare, this means that doctors can know the best course of treatment to prevent the worsening of someone’s disease or even prevent the onset of disease with early intervention. But many have also expressed concerns about machines doing the work of human doctors. However, a joint effort from both machines and doctors could significantly impact patient care. Prescriptive analytics for healthcare are still in its infancy.
3. Big Data in Healthcare
Data is emerging as the new fuel for growth; it all depends upon who can harness it best. In healthcare, the medical data about the patients and hospital operations is key to improving the quality of care. In this section, we will explore the role and impact of big data on the healthcare industry.
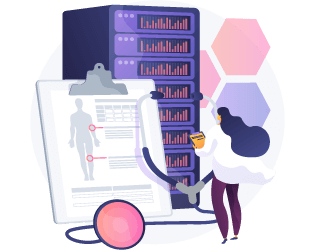
Big data analytics is one of the hottest buzzwords in the entire data analytics industry. In simple words, big data means data sets that are too large to be assessed using conventional analysis methods. The range of big data has been moving upward over the last few years, but today, it is generally accepted that data sets in the range of a few thousand terabytes to a few exabytes can be classified as big data.
The appearance of such large chunks of data in healthcare can be attributed to the rapid pace of digitization across the industry. Additionally, medical wearables such as Apple’s smartwatch and Fitbit, in addition to smart garments, also generate real-time data about users’ vitals. Furthermore, applications for medical billing, insurance claims, and electronic medical records are all brimming with users’ medical data. Increasing digitization will only increase the size of this data.
This heap of data undoubtedly contains a treasure trove of actionable insights about public health, which can be leveraged if this data can be processed. Advanced healthcare analytics solution for processing big data can help solve several industry problems and even tackle the country’s major health crises.
Let’s look at some of the problems healthcare analytics software can address using big data –
A. Opioid Abuse
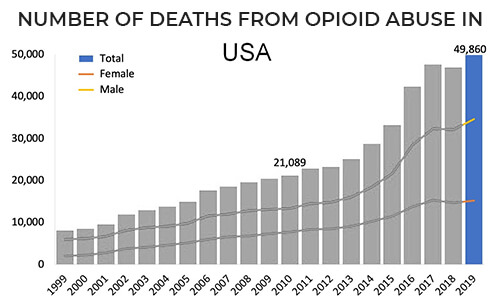
The number of deaths from opioid abuse in the United States resembles the figures one would normally see in an epidemic.
Estimates show that about 50,000 people died in 2019 from opioid abuse. That numb and nearly the same as US casualties during the Vietnam War. Medical researchers and doctors have agreed that this is a national health crisis that needs urgent government intervention. The solution to this problem might be hidden away in the large quantities of data the healthcare industry generates.
Healthcare data analytics solutions working with data acquired from insurance payers and pharmacies have identified hundreds of risk factors that might indicate if someone is likely to fall into opioid abuse. With this insight, doctors and therapists have been working on developing interventions to reach out to such individuals and prevent abuse.
B. Predictive Analytics
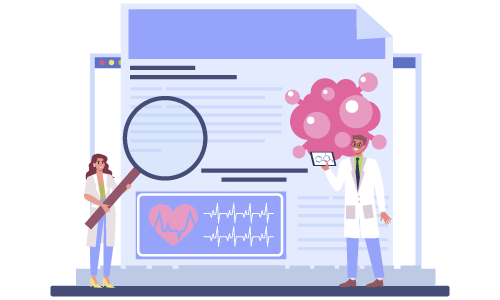
As mentioned earlier, this healthcare analytics approach can predict who might be at higher risk of certain conditions. Innovations in medical informatics have indicated the potential to assess millions of people’s historical and current medical data to recognize patterns of diseases. This approach to processing big data learns from millions of medical records to establish a threshold of acceptable risk.
When it recognizes a person to have crossed the threshold, it can predict if the person is likely to suffer from a disease for which the threshold was established. To be clearer, predictive analytics identifies people who are at higher risk of certain diseases based on their lifestyle and medical history. This is touted as a game changer for the industry as it can go a long way in helping to prevent chronic diseases and reduce the overall cost of care.
C. Patient Engagement
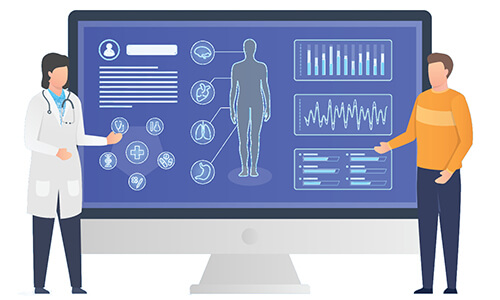
Patient engagement is one of the most underrated aspects of medical care that few people want to discuss. Many people aren’t capable of managing their care and hence need regular communication with their physician. This is especially true for people suffering from chronic diseases who need periodic check-ups and follow-up consultations.
It is not uncommon for patients to have doubts regarding their medication routines, dosage, tests, scans, or results. With the advent and proliferation of commercial wearables and other IoT-driven health tech, there is an avalanche of health data. Assessing this data gives important insights into the physical condition of the patients. Better yet, it can predict health risks for certain people and enable doctors to stage timely interventions.
As more and more providers use a patient engagement system, healthcare analytics services are bound to play an increasing role.
D. Hospital Staffing
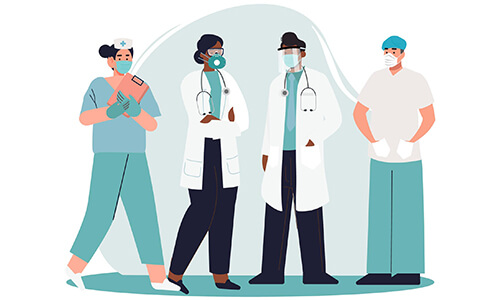
Large hospitals must often manage a large workforce consisting of doctors, nurses, orderlies, and other administrative staff. But the admissions rates often vary, and hospitals might find themselves either understaffed or overstaffed. While the former is bad for patient care, the latter is bad for the hospital as it would incur overtime expenses.
But big data accumulated over time from electronic health records of patients, insurance claims, billing activities, admissions, discharge, and so on can help alleviate the problem of efficiency in staffing. Administrators and staff managers can have a reliable idea about how much of the staff they would require at a given time and deploy the human resources optimally. So, using clinical analytics software for big healthcare data is a win-win for the hospital and the patients.
E. Identifying the Risk of Suicide
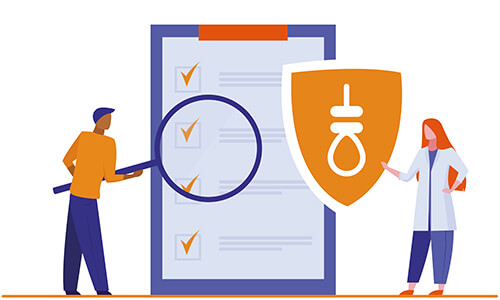
Various studies and estimates suggest that between 750,000 and one million people choose to end their own lives. Moreover, the same studies also revealed that more than a billion people are likely to commit self-harm at least once. This is shocking and unfortunate in today’s world, where mental health experts are available for help.
But big data has revealed that it is possible to combine information from electronic medical records and specialized questionnaires to identify the people at greater risk of suicide. Although this initiative is only in its initial stages and more data needs to be accumulated on its reliability, it might very well help hundreds of millions receive the desperately needed help.
F. Managing Epidemics and Pandemics
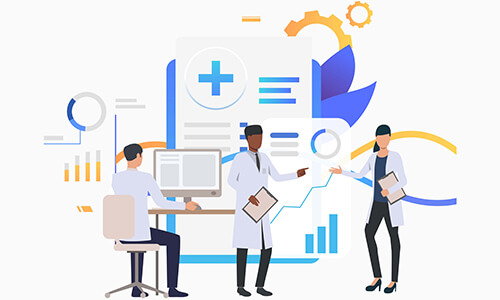
The recent Covid-19 pandemic was not the first epidemic and certainly won’t be the last. The coronavirus was a disease just like any other disease discovered and studied throughout the history of medical science. But the cures for the diseases we have today are the product of years of research and countless fatalities. But pandemics, by nature, spread fast and put countries’ healthcare systems under enormous strain. During such times, it becomes extremely difficult to study the nature of the disease and find a cure.
But big data analytics for healthcare did prove to be a useful weapon in the fight against Covid-19. Using this, medical researchers were able to identify the nature of the disease, how it spreads, conditions conducive to its spreading, the way it mutates, how fast it would mutate, how much it will spread, the fatality rate, common symptoms, demography of victims, and so on. Clinical analytics of healthcare big data of millions of people from the United States and around the world enabled experts to identify disease patterns and devise preventive measures to curb its spread.
This implementation of healthcare analytics solutions to relevant big data proved to be a potent tool for improving population health management policies. The industry was not prepared for this pandemic. But it was a wake-up call for governments and public health experts to take preventive measures. Big data analytics in healthcare could be an important tool for fighting future pandemics.
G. Chronic Disease Management
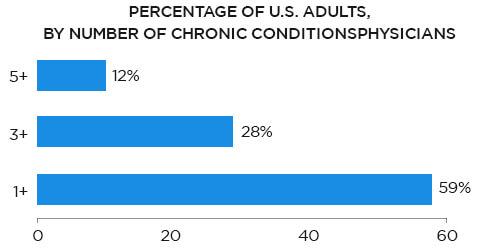
Chronic diseases last more than a few months and usually deteriorate over time. The most common ones in the United States include cancer, stroke, diabetes, and heart disease. Unfortunately, chronic diseases have become a major healthcare crisis in the country, with more than 40% of adults in America suffering from at least one.
While public health experts and policymakers are struggling to find a working solution, big data may have a way to alleviate the problem. By assessing various parameters such as personal health histories, medication history, the pattern of symptoms, frequency of clinical visits, and so on, it is possible to know who is at greater risk of hospitalization due to chronic diseases. This insight enables doctors to take a preventive approach to care. This would not only save lives and improve clinical outcomes, but it will also reduce the cost of care due to admissions.
Big data holds a treasure trove of solutions to address many health concerns. The right healthcare data analytics software can harness those to save lives.
4. Benefits of Data Analytics in Healthcare
We have seen what data analytics is and the various ways in which assessing large volumes of healthcare data can benefit all the stakeholders in the industry. It is important to understand big data’s role in the medical industry and how harnessing it would solve problems. In light of that, we will discuss the various benefits of implementing healthcare data analytics.
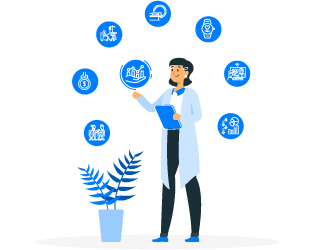
The potential applications of assessing big data the right way hold too many to be discussed all at once. Additionally, more and more insights continue to be discovered each year. It is no wonder that data analytics as an industry has received hundreds of millions in venture capital investment to support innovations. Entire companies have been built, and start-ups have grown over this.
Big data aside, assessing healthcare data generally holds numerous advantages for doctors, patients, and even insurance payers. Let’s explore some of those benefits in detail –
I. Potential for Personalized Treatment
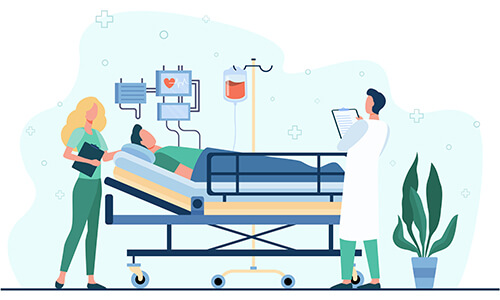
No two people are the same, and different people respond differently to the same treatments. While some people might get better with a course of treatment and resume their lives within a few days, others might not be so lucky. It depends on a person’s medical history, prevailing health, genetic predisposition, allergies, and other factors. In light of this, analytical tools in healthcare have progressed to the point where providers can assess a person’s innate body constitution and devise a personalized approach to treatment that is unique to each person.
Personalized medicine is one of the most talked about aspects of healthcare, and many consider it one of the big things to look forward to. It has created a buzz in medical circles and among many average people. Numerous studies have even suggested that it has the potential to revolutionize medicine as we know it. While mainstream medicine has followed the one-size-fits-all approach since its inception, personalized medicine might change that.
II. Reduced Cost of Care
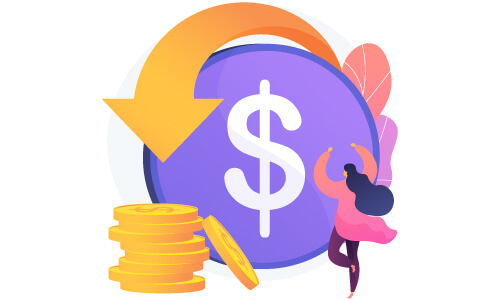
Hospitals, like every other company, ultimately depend on their revenues. While providing care might be the main priority, doing so in a profitable way is paramount. Advanced analytics for healthcare can assess operational and administrative data from everyday workflows at hospitals to identify which activities take up most of the resources. With this knowledge, the senior management can take steps to optimize things.
Aspects of healthcare like billing, coding, insurance claims, managing inventory, supply chains, vendors, and other operational overhead can be assessed for efficiency. Subsequently, healthcare IT analytics reveals important patterns about all the operations to highlight what can be done. This enables administrators to make informed decisions regarding optimization. One of the most popular solutions for this problem is healthcare automation. As the name suggests, it automates manual, repetitive tasks to improve speed and productivity and minimize errors. As a result, hospitals can experience a reduction in the cost of care, thereby increasing their revenues.
III. Better Patient Outcomes
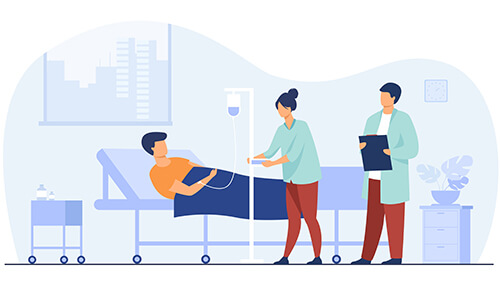
As mentioned, assessing large quantities of medical information reveals actionable insights about patient health and treatment. Electronic medical records, insurance claims, the usage of health plans, prescriptions, and other data points provide a treasure trove of information for medical analytics software to process and highlight the pain points.
Subsequently, this enables doctors to get a complete picture of a patient’s health and understand what kind of treatments might have a greater effect. This is especially true for chronic diseases, substance abuse, and other common illnesses. By assessing large amounts of historical data about the diseases and the patient’s response to the corresponding treatments, a healthcare data analytics solution can shed light on ways to improve treatments. This, in turn, will certainly result in better clinical outcomes for patients. Healthcare analytics solutions have been widely discussed as a way toward a value-based healthcare model.
IV. Improved Medical Imaging
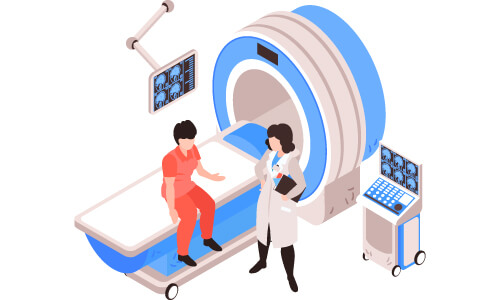
Medical imaging consists of X-rays, CAT Scans, MRI (Magnetic Resonance Imaging), CT scans, and so on. Assessing these images usually takes time, and doing so manually might result in some medical conditions being missed in their early stages. In some cases of cancer, the physicians missed it because the tumor was too small as it was in its early stages.
But healthcare analytics solutions powered by advanced technologies like artificial intelligence and machine learning can process many scans to learn to analyze scans faster. These systems learn from hundreds of thousands or even millions of medical scans to become increasingly better at detecting diseases.
V. Accelerate the Adoption of IoT
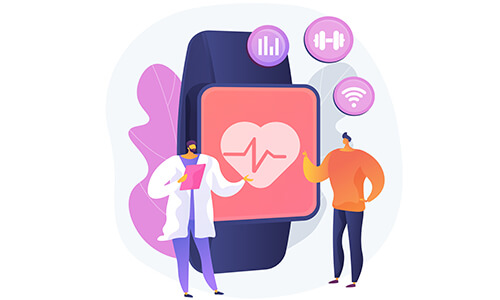
Wearable medical technology is fast becoming a fad with the average person, fitness enthusiasts, and professional athletes. As mentioned previously, gadgets like Apple’s Smartwatch, Fitbit, and other wearables can collect data on the users’ vitals in real-time. This information helps to know about the general physical condition. Vitals like an electrocardiogram, heart rate, body temperature, tracking sleep patterns, etc. It is estimated that hundreds of millions of people use such devices regularly.
Athletes use them to measure their performance scientifically and see where they need to improve regarding various parameters. Apple has teamed up with researchers to develop a solution to analyze heart rate and electrocardiogram to see if a user is at risk of a heart attack. This is just one example of data analytics applications with IoT-powered wearable devices.
The proliferation of healthcare data analytics platforms across the industry will accelerate the adoption of IoT technologies and personal medical devices. It could lead to a new approach to care.
VI. Remote Patient Monitoring
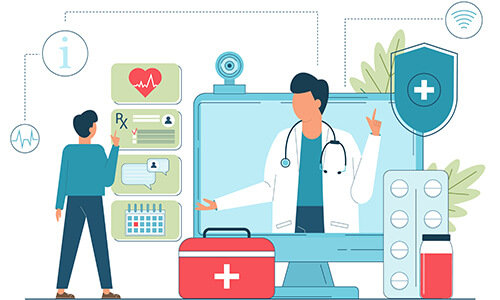
As the name indicates, remote patient monitoring (RPM) involves monitoring patients outside conventional clinical settings. Examples of this include home care and care of seniors in retirement communities. Assessing data gleaned from medical devices like an electrocardiogram, glucose monitors, and medical wearables helps to know if a person is at risk of any medical emergencies. That is because real-time health data acquired over time and analyzed appropriately highlights prevailing medical conditions without the person required to be in a clinic.
This could prove to be highly useful for senior care since it is estimated that there will be millions of seniors by the end of this decade and not enough caregivers. Remote patient monitoring fuelled by healthcare data analytics can bolster elder care in the country.
5. Challenges in Healthcare Data Analytics
Analyzing the data from the healthcare industry has demonstrated the potential to address many clinical and administrative challenges. We have seen the various uses of assessing data and all the benefits it can provide to doctors, patients, and even insurance payers. Yet, despite these advantages, implementing data analytics in healthcare isn’t that simple. In this section, we will discuss the challenges associated with it.
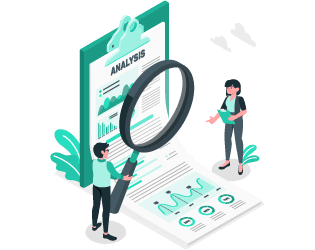
Here are some of the major challenges associated with analyzing healthcare data –
1. Storage
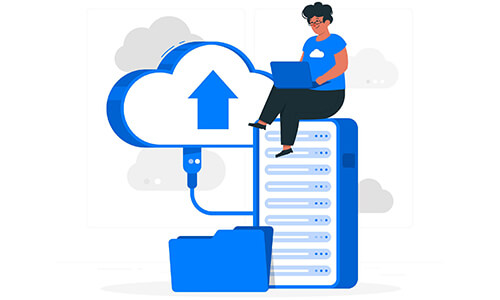
As mentioned earlier, the growing digitization of the healthcare industry can only mean an increase in the amount of data generated and exchanged. Moreover, the increasing popularity of IoT-powered medical wearables generates health data about hundreds of millions of people regularly. Medical data is being generated at an exponential rate with each passing year. So, the first thought that comes to mind is the efficient storage and management of this mound of data. The sheer hardware and network bandwidth requirements for this much information invariably mean that the organization can no longer store it all on-premises going forward.
That is where cloud computing in healthcare can help.
Many healthcare organizations are shifting their data to the cloud and using the services of third-party vendors. Most hospitals use integrated healthcare solutions for everyday operations and tend to use a hybrid approach to storage. However, the rate at which the amount of data continues to increase has raised many concerns in the medical and IT communities.
2. Data Scrubbing
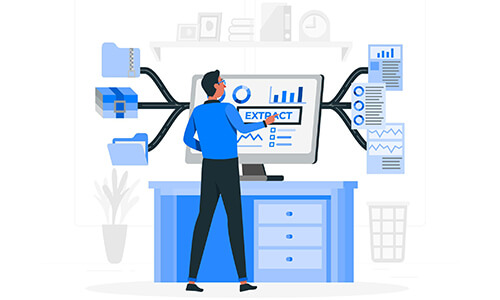
While the growing size of the data has raised concerns over its storage, the quality of that data is a major challenge that needs to be addressed. In this case, quality means the usability of the data for clinical analytics.
The healthcare data is collected from many disjointed sources like electronic records, insurance claims, health plans, medical billing, and even medical wearables. Data must be stored and cataloged in a certain way and verified for accuracy. It is kind of like arranging hundreds of books in a library in a systematic way. Moreover, the data must be accurate, relevant, and not corrupted. This ensures that health care data analytics programs can work effectively to produce the required insights. Arranging the data and ensuring that it is fit for analytical programs can be termed scrubbing the data.
The challenge with scrubbing is the sheer volume of it, coupled with its rapid growth. Conventional means of scrubbing have proven to be increasingly inefficient in light of the growing size of the datasets.
3. Cybersecurity
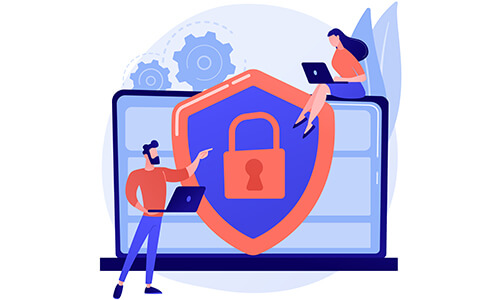
In the current digital age, cybercrime is a growing menace that has the largest corporations in the world investing millions in protecting their data. Hackers have been getting increasingly audacious in the attacks since there is enormous value for people’s data on the black market. In light of this, protecting healthcare data is a major priority for healthcare organizations. Given the growing size of the datasets, ensuring their security from unscrupulous individuals is becoming a challenge.
HIPAA-compliant solutions have become integral to health tech. Everything from medical records and practice management software to insurance verification, billing, and claims has to adhere to HIPAA regulations. In addition to cyberattacks, human error can also result in data loss. Incidents like theft of laptops, smartphones getting hacked, accidental sharing of credentials to the wrong people, or even corporate espionage can threaten digital security.
So, it is important to incorporate the latest versions of the software in use, coupled with the best anti-virus software, and also train the staff on ensuring the security of their devices.
4. Data Sharing
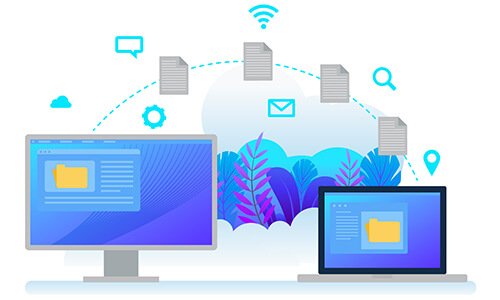
Analyzing medical data efficiently invariably entails sharing it. Patients are bound to visit different providers throughout their lifetime and won’t have the luxury of getting all their care at the same clinic. Moreover, doctors, too, would have to handle patients with their records stored elsewhere. So, patients’ medical records must be shared seamlessly among various providers for analytics and care.
But given the disparate health systems, healthcare interoperability sometimes gets challenging since electronic data exchange isn’t always easy. The development of APIs (Application Programming Interface) and standards like the FHIR (Fast Healthcare Interoperability Resource) are some of the industry’s steps to ensure smooth sharing. But as mentioned before, since the sheer volume of data is growing and innovations bring in newer formats, the challenge of sharing is bound to evolve.
5. Data Capture
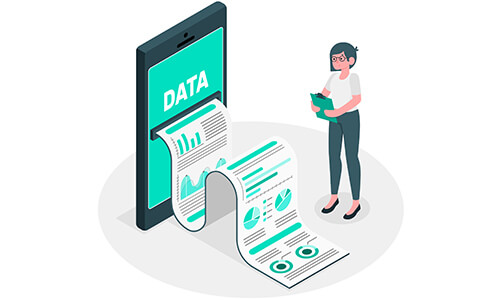
As mentioned earlier, the ongoing digitization of the healthcare industry would generate data from multiple sources. Moreover, the rapid adoption of remote healthcare technologies coupled with medical wearables gaining popularity only adds to the growing data sources for the healthcare industry. In light of this, capturing relevant, actionable, scrubbed (mentioned above), and up-to-date data is proving to be a challenge.
Doctors need patients’ data to be stored in a certain way and format. In the absence of this, the usability of the data diminishes. This eventually impacts clinical decision-making and also tends to lead to redundancy. In some cases, it might also lead to adverse medical outcomes. This eventually costs the providers, patients, and insurance payers more and becomes a lose-lose situation for everyone involved. In addition to clinical activities, incongruent data also could affect administrative workflows.
6. Querying
Organizations need to query the data available to use it. Querying it would fetch organizations what they need and help them make decisions accordingly. But having large datasets garnered from disparate can make querying extremely challenging.
Most healthcare organizations struggle with interoperability problems due to data siloes created by disjoint healthcare solutions. If different parts of a dataset are stored in multiple disjoint data siloes from different systems, the usability of the data becomes challenging. Clinicians would not obtain a complete picture of the patient’s medical condition and background, which impacts the medical outcomes.
One of healthcare analytics solutions’ biggest challenges is sorting and categorizing the data in a way that is of use for clinical operations.
6. How Did Data Analytics Help Fight Covid-19?
The Covid-19 pandemic will always be remembered as one of the biggest tragedies of this generation. It devastated entire economies, triggered a financial recession, shut down businesses, and claimed millions of lives. While rapid medical research and vaccine development saved us from this pandemic, data analytics is one of the unsung heroes in this fight. In this section, we explore how assessing medical data helped us shorten this crisis.
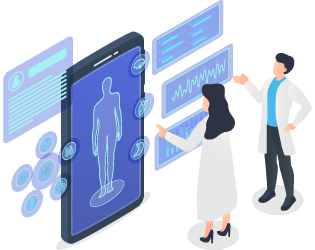
It began as a viral outbreak in the Wuhan Province of China in late 2019 and quickly spread far and wide. There were warnings, but before governments could know more and mount a full-scale response, it had already spread across the globe. The Covid-19 pandemic caused untold economic upheavals in every country it touched. Hundreds of millions lost their jobs, and several industries like tourism, aviation, retail, and others were badly affected.
Researchers worldwide raced to develop a vaccine to protect humanity from this menace during this time. While progress can be attributed to the work done in research laboratories, we cannot ignore the work done at computing centers. These places crunched the medical information from around the world about the coronavirus and its effects. By assessing various parameters of the virus, its nature, symptoms, and mode of transmission, clinicians could assist governments in implementing policies to curb the spread of infection.
Let’s explore in detail how healthcare data analytics platforms helped protect us from the worst of the pandemic –
A. Identifying Patients at Risk
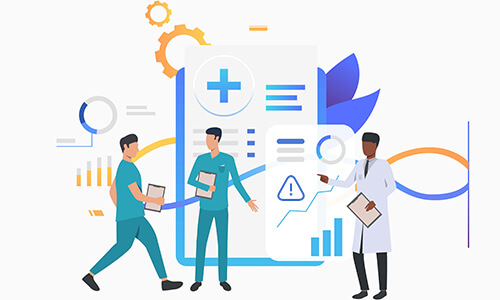
This is one of the most exciting applications of data analytics in healthcare. During the pandemic, it was well-known that certain people were at greater risk of contorting the virus. These included people over 60 years of age, people suffering from breathing problems like asthma or bronchitis, heart disease, pregnant women, infants, and toddlers under nine years of age.
In addition to people with such health problems, individuals whose jobs involved being in crowded places were also at greater risk of catching the virus. These people include military personnel, hospital staff, pilots, cabin crew, etc. Numerous such parameters made it possible to pinpoint people at increased risk of getting infected, and the government could then issue guidelines to such people to take appropriate precautions.
Analyzing large datasets containing medical information of people helps to reveal such insights. Based on medical history and demographic information, clinicians could find out the people at greater risk.
B. Predicting Increase in Infections
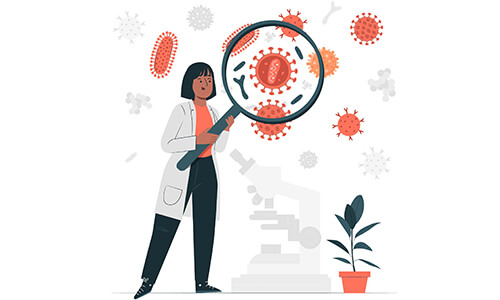
The rate of spread of infections differed from location to location. Numerous cities and towns across the globe fought varying surges in Covid-19 infections based on multiple parameters like population density, availability of care, capacities of hospitals and clinics, number of people vaccinated, restrictions imposed by local government, and so forth.
Assessing all of this data reveals patterns and possibilities of how much a virus could spread in which community. For example – a place like New York City is likely to suffer from surges of greater intensity than compared to smaller towns in the Central United States. That is because a place like New York City is densely populated, likely has a lower ratio of people to caregivers, and is far more likely to experience crowding. So, based on reliable parameters, analytics solutions can accurately predict where surges can happen.
This insight helps policymakers and public health experts take appropriate measures to contain the spread of infection. This is a powerful tool that some governments across the world have deployed and has helped them better manage the Covid-19 situation.
C. Projection of Resources Necessary
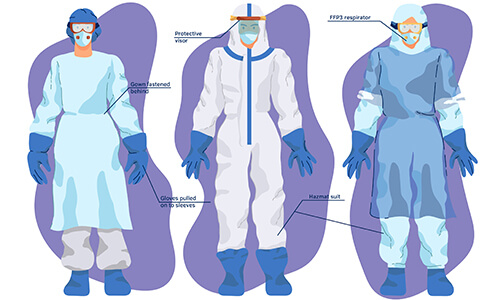
The initial days and months of the pandemic saw many countries struggling with vital supplies like face masks, face shields, and personal protection equipment (PPE). The virus forced entire countries into curfews and lockdowns, affecting supply chains, transportation, distribution, and manufacturing. Additionally, oxygen became a scarce commodity for which hospitals and clinics struggled. Some healthcare organizations in a few developing nations had to turn away patients due to a lack of medical-grade oxygen.
In light of this need, data analytics can help to obtain a reliable estimate of the requirement of the essential goods mentioned above. Based on factors like the number of people with chronic disease and respiratory problems in a particular location, it is possible to have projections of the number of people likely to get infected and the quantity of equipment that would be needed.
This helps the government and public health experts make informed decisions about the production and acquisition of essential equipment to help curtail the spread of infection.
D. Better Reporting
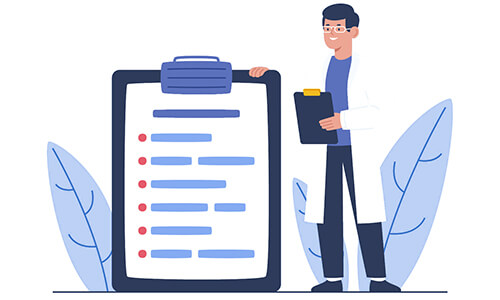
When the pandemic was at its peak, hospitals were overloaded, the medical staff was stretched to the breaking point, and there were widespread shortages of all kinds. The healthcare systems of all countries, including the United States, simply were unprepared for the avalanche of patients crowding hospitals. Medical organizations had to deal with massive caseloads, in addition to dealing with non-covid ailments which normally occur. Amid this chaos, medical reporting was falling short because everyone was over-stretched.
In light of this, leveraging healthcare data analytics solutions helped to bridge this gap in reporting abilities. Assessing large amounts of clinical and administrative information enables the management to compile detailed reports stipulated by government regulations. These further enable public health officials to better understand the situation and mobilize the necessary response.
This also has the other effect of facilitating better medical records management during medical crises.
7. The Role of Data Analytics in Value-Based Care
The prevailing healthcare model invariably leads to high costs, which are being questioned by policymakers, insurance payers, and common people alike. In light of the growing costs of care, there has been a push for a value-based approach to care. This section will explore how healthcare analytics solutions can help usher in value-based care.
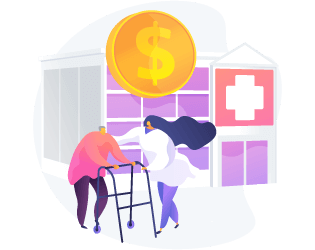
The current model of care involves patients getting billed for every consultation, test, scan, and prescription. It is called the fee-for-service model and, as the name suggests, charges for every medical service provided. It incentivizes providers to offer more services and rewards the same, without emphasis on the medical outcomes for patients. Needless to point out, it invariably leads to higher care costs, making it more and more difficult for people to avail themselves of it.
On the other hand, a value-based model of care focuses more on the clinical outcomes for the patients, as opposed to the number of medical services. In this model, providers are paid based on their patient’s health situation after the treatments, not the number of consultations, scans, etc. Analyzing population medical data reveals many things that providers and payers can use to help reduce the cost of care.
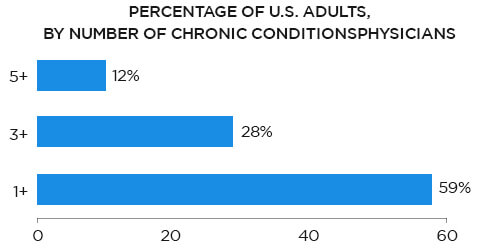
Parameters like individual medical histories and broader population health metrics can fuel data analytics when finding ways to fill in gaps in clinical processes. There are numerous reasons why patients might have undesirable medical outcomes. These include medication errors, lack of timely access to electronic medical records misdiagnosis, insufficient patient engagement, treatment delays due to administrative holdups, insufficient insurance coverage, and many more. Assessing historical and current information about clinical and administrative processes in healthcare makes it possible to empower clinicians with better tools for clinical decision support. This would, in turn, also engender a more collaborative approach to care where multiple providers would coordinate their efforts to treat patients.
Such an approach would go a long way in improving the outcomes for people suffering from chronic diseases. More than 40% of adults in America suffer from at least one chronic disease, which also accounts for one of the biggest expenditures on healthcare. People with such illnesses often require regular doctor visits, frequent tests, medication, and so forth. The current fee-for-service model of medical reimbursements would result in a massive bill for people with chronic diseases.
But analyzing healthcare big data with the appropriate parameters would help streamline clinical workflows and optimize the number of clinical visits. This results in effective clinical decision support, better treatments, good doctor-patient engagement, and improved patient outcomes. Moreover, this approach would also reduce the cost of care by a significant margin, allowing more Americans to access the care they need.
Big data can help providers determine what parts of the population are at increased risk of certain diseases. The parameters needed for such a deduction include:
- Genetic predisposition
- Ethnic population health indicators
- Medical history
- Access to care
- Other aspects are specific to that part of the population
This gives a reliable picture of the existing health situation of certain groups of people and enables doctors to make better clinical decisions.
In this way, assessing large quantities of data about healthcare reveals actionable insights that clinicians can use to identify gaps in their services. When tiny problems add up over time, they tend to lower the quality of care than it could have been, impacting patient outcomes. However, the insights gained from analyzing data about healthcare could help iron out those kinks and improve medical care for everyone.
This is one of the biggest ways in which data analytics could help the shift towards a value-based model in healthcare, as opposed to the current fee-for-service model. It would lower the costs, make care more accessible, and improve the outcomes. It’s a win-win for all stakeholders involved.
8. How Does Data Help in Improving Clinical Outcomes?
We have discussed the role of data in healthcare extensively. Assessing data has helped improve the efficiency and productivity of more or less every industry where it has been deployed. In healthcare, it improves the productivity of the administrative activities and the outcomes of the care provided to patients. In this section, we will explore how that would happen.
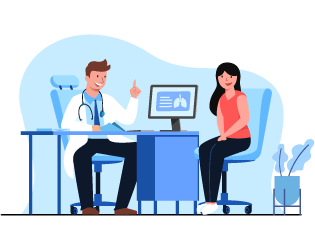
People’s electronic health records contain their entire medical histories. This includes basic information like height, weight, and age, as well as immunization, allergies, medication history, previous diseases and corresponding treatments, history of substance abuse (if any), accidents, and so on. This information is important for doctors to make informed decisions about care.
For example, if a person with a history of heart disease comes in with an illness, his or her treatment or medications might differ from those without any heart disease. This is because people with existing conditions might respond differently to treatments than those without any such conditions. The same holds for people with other diseases (especially chronic ones).
In addition to health histories, people’s ethnicities also play a part in their health situation. It’s not exactly new knowledge that not everyone enjoys equitable access to healthcare. Ethnic minorities don’t have the same access to care as others do. Moreover, people of this demographic are also more likely to follow lifestyles that would increase the chances of diseases and substance abuse. This is partly fueled by poor access to quality education and generally poorer nutrition on average.
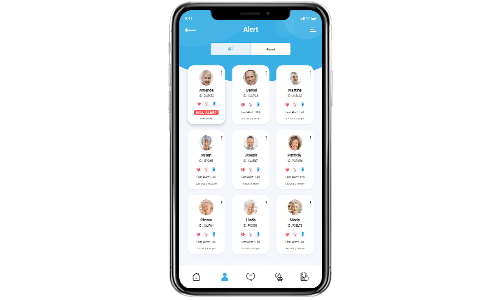
These factors serve as data points and make the overall information about public health. This information, coupled with electronic health records and personal medical histories, enable data analytics solutions to identify the gaps, discrepancies, and inequities in existing clinical workflows. Subsequently, hospital management, policymakers, and insurance payers can change existing systems and practices.
The most exciting part of data analytics and assessing all these factors is predicting who is at greater risk. Based on the parameters mentioned above, healthcare data assessment can highlight which persons need hospitalization shortly or might be more likely to suffer from heart attacks. Based on this, doctors can carry out early-stage interventions for these people and can prescribe immediate treatments to avert a worsening of health. This would involve medications, changes to lifestyle, diet, and other things to improve the patient’s health. Doing so would prevent hospitalization or worsen health.
This preventive approach to care is better than the reactive approach, where people go to the doctor only after they experience serious symptoms. Such a data-driven approach to care has better outcomes and reduces the cost of care. This is one of the most potent ways to transform healthcare using data.
To sum it up, a predictive approach to care would reduce the instances of chronic diseases, improve clinical outcomes, lower the cost of care, and even has the potential to extend the average life expectancy. It is a win-win for all stakeholders – the patients, providers, and insurance payers.
9. How Can Data Analytics Improve Patient Engagement?
Patient engagement is one of the most undervalued aspects of care. Numerous surveys and research have repeatedly demonstrated a direct correlation between good patient engagement and favorable clinical outcomes. This section will explore how healthcare data can be leveraged to boost engagement.
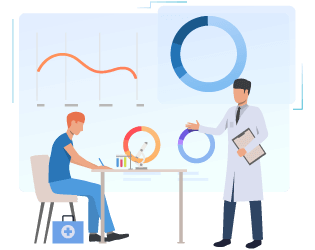
Patients need to be an active part of the entire care journey. Gone are the days when the doctor prescribed medications and the patients just took them. As the country faces an alarming rise of chronic diseases coupled with the growing costs of care, good patient engagement has empowered people to take charge of their care and actively participate in their recovery. This involves making changes to lifestyle, adhering to medication dosage, following the recommended diet, and being regular for follow-up consultations.
Good doctor-patient rapport encourages the patients to lead healthier lives and take their health seriously. It is known to make a difference in the lives of people suffering from chronic diseases like diabetes, cancer, heart disease, and so forth.
Data analytics has helped providers alleviate some of the biggest challenges in engaging with patients. One of them is appointment no-shows.
Not showing up to appointments on time is one of the biggest reasons for worsening existing conditions. People prioritize their work and social life over going to a doctor and tend to postpone multiple times. For people suffering from chronic diseases, appointment no-shows can majorly impact their long-term health and medical outcomes.
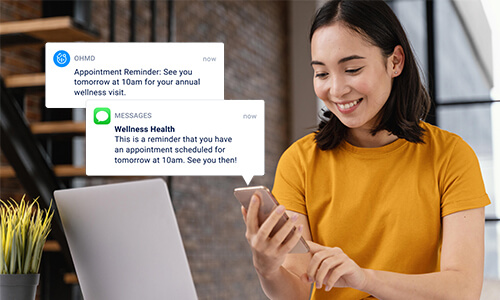
Data analytics and automation help providers identify the people who are likely not to attend appointments. Additionally, healthcare automation solutions integrated with electronic medical records and hospital systems can send appointment reminders through text messages and even phone calls. Moreover, these solutions can also help the hospital staff coordinate with the patients and easily reschedule an appointment at a later date.
Some of the more advanced solutions also offer the alternative of virtual consultations in case of missed appointments. In addition to people with chronic diseases, this is extremely helpful for people who have just been discharged after a serious illness. For such people, follow-up appointments are vital for ensuring that they are coping and would make a full recovery. During each successive follow-up, the doctor would check the patient’s vitals and conduct tests to ascertain good health. Any deviance from expected results would result in appropriate treatments or medications. But what is necessary is the timeliness of the patients’ visits, which data analytics can help improve through more effective patient engagement.
Another aspect of patient engagement is pill reminders. These are applications that can be downloaded by anyone who needs it. As the name indicates, such applications remind patients if it’s time to take their medication. Users can enter the names of the medication prescribed to them and the timings of the dosages. The application automatically reminds users of the time for taking specific pills/injections/syrups. This is another form of tech-driven patient engagement that helps to improve clinical outcomes.
10. Role of Data in Pharmaceuticals
Pharmaceuticals are integral to healthcare and are the backbone of the modern medical industry. Pharmaceuticals conduct research and develop cures for a wide range of diseases, which are accessible to people through providers. This section will explore how data can help accelerate pharmaceutical processes.
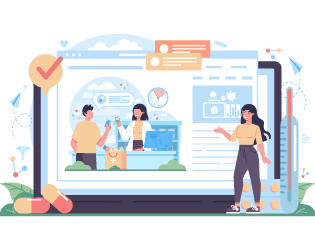
Pharmaceuticals need empirical data from research to develop medication for every disease imaginable. Whether it is something as benign as cold, headache, or aches to serious illnesses like malaria, typhoid, and cancer, data propels pharmaceutical companies’ research. Let’s look at the role of data –
I. Drug Discovery
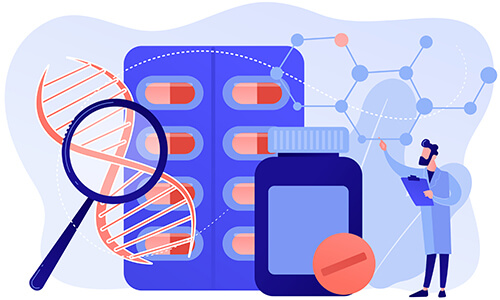
It takes well over a billion dollars to bring a new drug into the market, and the money goes into research, clinical trials, manufacture, and distribution. However, some pharmaceutical companies have even begun harnessing big data in healthcare to accelerate drug discovery. This is done by accessing information derived from research papers, trials, findings, and other medical publications. This insight would also empower predictive analytics algorithms with the right parameters for accelerating drug discovery.
II. Clinical Trials
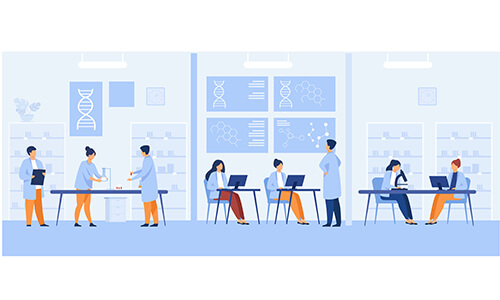
Trials for every drug require the right people to act as volunteers, and these people are selected carefully based on strict parameters. Healthcare big data can help accelerate this process by speeding up the search for the ideal trial candidates. This is based on information garnered from EMRs, insurance plans, etc.
III. Risk Assessment
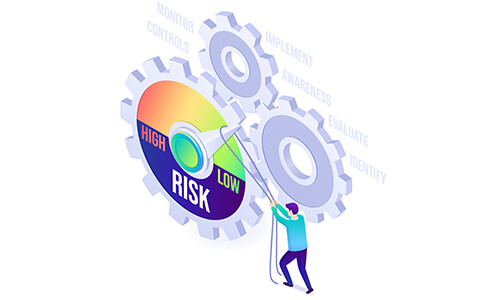
Drugs developed for every type of complication might not sit well with everyone, and there are bound to be a few people for whom certain drugs might have adverse reactions or not be as effective. Assessing big data in healthcare helps researchers predict such reactions and sometimes even identify people likely to experience undesirable effects, and this knowledge helps them take steps to mitigate risk.
Conclusion
Data is the fuel that propels growth and productivity in all industries, and Healthcare can work wonders for patient care while also bringing down costs. As the industry continues to adopt digital technologies every year, the amount of data to be processed is bound to increase. So, healthcare analytics solutions need to evolve to meet the growing demand.
OSP is a trusted healthcare software development company that delivers bespoke solutions as per your business needs. Connect with us to hire the best talents in the industry to build enterprise-grade software.
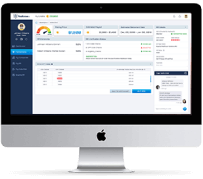
How can we help?
Fill out the short form below or call us at (888) 846-5382
Discuss Your Project Handover with a team of expert Book a free consultation arrow_forward
About Author
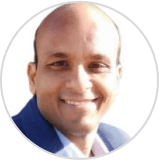
Written by Riken Shah linkedin
Riken's work motto is to help healthcare providers use technological advancements to make healthcare easily accessible to all stakeholders, from providers to patients. Under his leadership and guidance, OSP Labs has successfully developed over 600 customized software solutions for 200+ healthcare clients across continents.